Research Area C - Intelligent sensor network, robustness, data management, ecosystem model, and deep learning
Area C gathers cross-cutting research projects comprising data acquisition through an intelligent, energy-autonomous and robust sensor network. It assures a comprehensive and quality-assured database, process-based ecosystem modelling and deep-learning assessment.
C1 Wireless, energy-autonomous and energy-aware embedded sensor systems
C1.1 Energy autonomy and energy awareness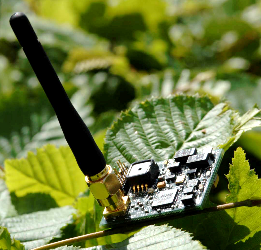
We will perform research on specific needs for all distributed sensors (from areas A and B) creating a number of innovations for embedded autonomous sensor systems. All sensors will be equipped with ultra-low power electronics and communication capabilities to go beyond their pure sensing function, and to enable them as active and intelligent sensor nodes in our ECOSENSE network. Self-test features and energy awareness will be incorporated to improve a sensor node’s performance over time.
Open positions: currently there are no open positions in this subproject.
C1.2 Ultra-low power and long-range radios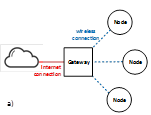
Wireless communication between individual sensor nodes and central sensor hubs will be provided, by using newly established communication networks (e.g. narrow-band Internet-of-Things or NB-IoT), as well as specially tailored low-power local radio (e.g. LoRa). The energy supply of such widely distributed sensor nodes has to happen either via energy harvesting from thermal, solar, or mechanical ambient energy, or via passive remote sensing approaches similar to today’s RFID technology.
Open positions: currently there are no open positions in this subproject.
PIs: Prof. Dr. Stefan J. Rupitsch and Prof. Dr. Leonhard Reindl
C2 Antibiofouling and Antisoiling Coatings
We will investigate how sensor nodes, such as soil probes and leaf sensors, being in direct contact to the environment can be designed to be long-living and stable by preventing fouling processes, such as the accumulation of dirt or bacterial growth. This requires tailored surface structures and antifouling coatings, whose specification strongly depends on sensor location. In soil, they are exposed to long wet phases, while in air they are exposed to large temperature and humidity variations, or simply to the accumulation of dirt.
Open positions: currently there are no open positions in this subproject.
PIs: Prof. Dr. Jürgen Rühe, Dr. Oswald Prucker, Prof. Dr. Peter Woias, Dr. Laura Comella, Prof. Dr. Ulrike Wallrabe
C3-INF Data management
We will generate, manage, adapt, and extend a joint database for all project data, securing homogenised formats, storage, selection, and accessibility by all groups. As ECOSENSE relies on data interoperability within and between its projects, the challenge lies in managing easy data access using existing data formats where appropriate, and creating new research data formats where necessary. We will use a central platform based on AQUARIUS for joint data storage, management of all ECOSENSE projects, and provide services for data exchange and shared data analysis tasks.
Open positions: currently there are no open positions in this subproject.
C4 Deep-learning and process-based models for simulating carbon and water fluxes across scales
C4.1 Process-based model simulations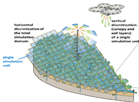
We will carry out process-based model simulations with the objectives to 1. Improve model representations of physiological processes, water and carbon balance, and 2. Optimize spatio-temporal resolution of sensor-based monitoring. Therefore, the 2D LandscapeDNDC model will first be analyzed, extended and/or modified based on results obtained with deep learning tools (from C4.2). Afterwards, model results will be compared with different numbers and setups of sensors to improve measurement efficiency.
Open positions: currently there are no open positions in this subproject.
PIs: PD Dr. Ralf Kiese and Dr. habil. Rüdiger Grote (KIT)
C4.2 Deep learning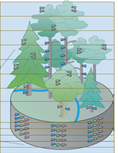
Using deep-learning algorithms, the plethora of data will be efficiently evaluated to distinguish between important and redundant data. The aim is to provide sufficient spatio-temporal resolution and save sensor node energy and reduce redundant data accumulation. Thereby, deep learning and process simulations interact with the sensor network through i) data assimilation from the sensor network and ii) adjustment and optimization of the measuring design based on simulated outputs and predictions
Open positions: currently there are no open positions in this subproject.